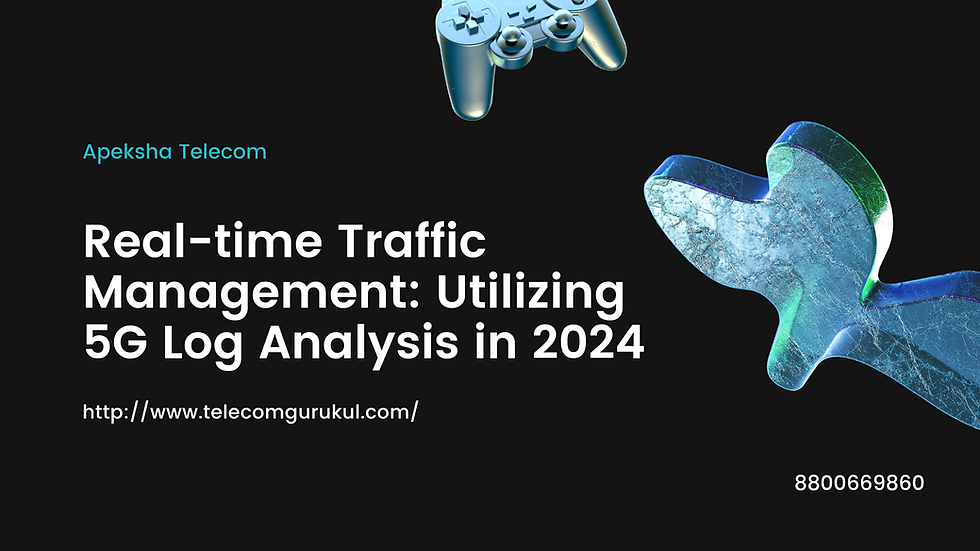
In the dynamic landscape of telecommunications, the efficient management of network traffic is crucial for ensuring optimal performance, reliability, and user experience. With the advent of 5G technology, the volume and complexity of network traffic have increased exponentially, presenting new challenges and opportunities for traffic management. In this blog, we delve into the realm of real-time traffic management and explore how organizations can leverage 5G log analysis to optimize network traffic in 2024. From understanding the importance of real-time traffic management to implementing advanced log analysis techniques, this comprehensive guide offers insights into strategies for enhancing network efficiency and responsiveness.
Table of Contents
Introduction
Understanding Real-time Traffic Management
Challenges in 5G Network Traffic Management
Importance of 5G Log Analysis
Advanced Log Analysis Techniques
Real-time Traffic Management Strategies
Conclusion
Introduction
In today's hyper-connected world, the efficient management of network traffic is essential for delivering seamless connectivity and meeting the growing demands of users. With the rollout of 5G technology, the volume, variety, and velocity of network traffic have reached unprecedented levels, posing significant challenges for telecom operators and network administrators. Real-time traffic management is the key to addressing these challenges and ensuring optimal network performance, reliability, and security.
Understanding Real-time Traffic Management
Understanding real-time traffic management involves grasping the intricacies of continuously monitoring and optimizing network traffic as it occurs. This proactive approach ensures efficient resource allocation, minimizes congestion, and maintains a consistent user experience. Real-time traffic management encompasses various techniques such as traffic shaping, load balancing, and dynamic routing to adapt to changing traffic patterns and demands effectively.
Challenges in 5G Network Traffic Management
The transition to 5G introduces new complexities and challenges in managing network traffic effectively:
Spectrum Fragmentation
Spectrum fragmentation refers to the division of available radio frequency spectrum into numerous smaller frequency bands in 5G networks. This fragmentation occurs due to the use of diverse frequency bands, including low, mid, and high bands, to support different 5G services and applications. Spectrum fragmentation poses challenges for network operators in efficiently allocating and managing spectrum resources, as it requires sophisticated coordination and optimization strategies to ensure optimal performance and coverage across all frequency bands.
Massive IoT Connectivity
Massive IoT connectivity refers to the widespread deployment and interconnection of Internet of Things (IoT) devices within 5G networks. This entails connecting a vast number of IoT devices, ranging from sensors and actuators to smart appliances and industrial equipment, to enable diverse applications such as smart cities, industrial automation, and environmental monitoring. Managing massive IoT connectivity involves addressing scalability, reliability, and security challenges associated with supporting a large number of devices and accommodating varying traffic patterns and data requirements. Additionally, optimizing network resources and implementing efficient communication protocols are essential to ensure seamless connectivity and efficient operation of IoT applications within 5G networks.
Ultra-reliable Low-latency Communication (URLLC)
Ultra-reliable low-latency communication (URLLC) is a key feature of 5G networks that enables highly reliable and low-latency communication for mission-critical applications. URLLC is designed to support services and applications that require ultra-low latency, high reliability, and deterministic communication, such as industrial automation, autonomous vehicles, remote surgery, and critical infrastructure monitoring. By guaranteeing stringent requirements for latency, packet loss, and reliability, URLLC ensures that time-sensitive and mission-critical data is transmitted with minimal delay and maximum reliability, enabling real-time control and response in demanding scenarios.
Importance of 5G Log Analysis
The importance of 5G log analysis lies in its ability to provide invaluable insights into the performance, behavior, and health of 5G networks. By analyzing the logs generated by network devices, applications, and infrastructure components, organizations can gain a comprehensive understanding of network operations, traffic patterns, and potential issues. This enables proactive monitoring, troubleshooting, and optimization of 5G networks to ensure optimal performance, reliability, and user experience.
5G log analysis facilitates:
Performance Monitoring: By analyzing log data, organizations can monitor key performance metrics such as throughput, latency, packet loss, and network utilization in real-time. This allows them to identify performance bottlenecks, optimize resource allocation, and ensure efficient network operation.
Anomaly Detection: Log analysis enables the detection of anomalies or deviations from normal network behavior, such as unusual traffic patterns, configuration errors, or security incidents. Early detection of anomalies allows organizations to take corrective action promptly, minimizing the impact on network performance and security.
Troubleshooting and Root Cause Analysis: When network issues arise, log analysis provides valuable information for troubleshooting and root cause analysis. By correlating log data from different sources, organizations can pinpoint the underlying cause of issues and implement targeted solutions to resolve them effectively.
Capacity Planning and Optimization: By analyzing historical log data, organizations can identify trends and forecast future network capacity requirements. This facilitates proactive capacity planning and optimization, ensuring that network resources are allocated efficiently to meet current and future demands.
Security Monitoring and Threat Detection: Log analysis plays a crucial role in security monitoring and threat detection. By analyzing logs for signs of suspicious activity, unauthorized access attempts, or security breaches, organizations can detect and respond to security threats in real-time, protecting their network infrastructure and sensitive data.
Advanced Log Analysis Techniques
Advanced log analysis techniques enable organizations to extract actionable insights from 5G log data:
Machine Learning
Machine learning plays a pivotal role in 5G log analysis, offering advanced techniques for extracting actionable insights from the vast amount of log data generated by 5G networks. By leveraging machine learning algorithms, organizations can enhance their capabilities in monitoring, diagnosing, and optimizing network performance. Here's how machine learning is utilized for 5G log analysis:
Anomaly Detection: Machine learning algorithms can automatically detect anomalous patterns or deviations from normal behavior in 5G log data. This enables early identification of network issues, such as abnormal traffic patterns, equipment failures, or security breaches, allowing organizations to take proactive measures to mitigate risks and maintain network integrity.
Predictive Maintenance: Machine learning models can analyze historical log data to predict potential equipment failures or performance degradation in 5G networks. By identifying early warning signs and patterns indicative of impending failures, organizations can schedule preventive maintenance activities, minimize downtime, and optimize network reliability.
Traffic Pattern Analysis: Machine learning techniques can analyze log data to identify patterns and trends in network traffic, such as peak usage periods, traffic spikes, or recurring patterns. This information helps organizations optimize resource allocation, capacity planning, and traffic management strategies to ensure efficient network operation and performance.
Root Cause Analysis: Machine learning algorithms can facilitate root cause analysis by correlating log data from different sources and identifying the underlying causes of network issues or performance degradation. This enables organizations to diagnose complex network problems more accurately and expedite the troubleshooting process, reducing mean time to resolution (MTTR) and improving overall network reliability.
Resource Optimization: Machine learning models can optimize resource allocation and utilization in 5G networks by analyzing log data and predicting demand patterns for network resources, such as bandwidth, spectrum, and computing resources. This allows organizations to dynamically allocate resources based on real-time demand, improve network efficiency, and enhance user experience.
Real-time Traffic Management Strategies
Effective real-time traffic management requires a combination of proactive monitoring, dynamic traffic shaping, and intelligent routing:
Dynamic Traffic Shaping
Dynamic traffic shaping is a network management technique that involves dynamically controlling the flow of traffic in a network to prioritize certain types of traffic over others based on predefined rules or policies. This allows organizations to optimize network performance, prioritize critical applications or services, and ensure a consistent quality of service (QoS) for users.
In dynamic traffic shaping:
Prioritization: Traffic shaping algorithms classify incoming traffic into different categories based on factors such as application type, protocol, or source/destination address. Priority levels are assigned to each category, allowing organizations to prioritize critical traffic, such as voice or video calls, over less time-sensitive traffic, such as file downloads.
Bandwidth Allocation: Dynamic traffic shaping allocates available bandwidth among different traffic categories based on their priority levels. Higher priority traffic is allocated more bandwidth to ensure timely delivery and minimal latency, while lower priority traffic may be throttled to prevent congestion and maintain overall network performance.
Adaptive Policies: Dynamic traffic shaping adapts its traffic management policies in real-time based on changing network conditions, traffic patterns, and user demands. This allows organizations to respond dynamically to fluctuations in network traffic, ensuring optimal performance and responsiveness even during peak usage periods or unexpected events.
Quality of Service (QoS) Enforcement: Dynamic traffic shaping enforces quality of service (QoS) policies to guarantee a certain level of performance for critical applications or services. By prioritizing high-priority traffic and limiting the bandwidth or resources allocated to lower-priority traffic, organizations can maintain QoS levels and meet service level agreements (SLAs) with customers or users.
Load Balancing
Load balancing is a network management technique used to distribute incoming network traffic across multiple servers, devices, or resources to ensure optimal utilization, maximize throughput, and enhance reliability. The goal of load balancing is to prevent any single server or resource from becoming overwhelmed with traffic, thereby improving overall performance and availability of the network.
In load balancing:
Traffic Distribution: Load balancers monitor incoming network traffic and distribute it evenly across multiple servers or resources based on predefined algorithms or policies. This ensures that no single server or resource is overloaded, preventing performance degradation and ensuring a consistent user experience.
Scalability: Load balancing allows organizations to scale their infrastructure horizontally by adding more servers or resources to handle increasing traffic load. As traffic grows, additional servers can be added to the load balancer pool, enabling the network to accommodate higher demand without impacting performance or availability.
Fault Tolerance: Load balancers enhance fault tolerance and reliability by automatically rerouting traffic away from failed or unresponsive servers. In the event of a server failure or outage, the load balancer redirects traffic to healthy servers, ensuring uninterrupted service and minimizing downtime for users.
Session Persistence: Some load balancers support session persistence or sticky sessions, which ensure that requests from the same client are consistently routed to the same server. This is particularly useful for applications that require session continuity, such as e-commerce websites or web applications with user sessions.
Health Monitoring: Load balancers continuously monitor the health and performance of servers or resources in the pool by periodically sending health checks or probes. If a server is detected to be unhealthy or underperforming, the load balancer stops directing traffic to that server until it becomes available again.
Conclusion
In conclusion, real-time traffic management is essential for optimizing network performance, reliability, and security in 5G networks. By leveraging advanced log analysis techniques and implementing dynamic traffic management strategies, organizations can adapt to changing traffic conditions, minimize congestion, and deliver a seamless user experience. As we move forward in 2024 and beyond, the importance of real-time traffic management and 5G log analysis will continue to grow, driving innovation and enabling the next generation of connected services.
Internal URLs:
Apeksha Telecom's training programs: https://www.apekshatelecom.com/training
Apeksha Telecom's placement assistance: https://www.apekshatelecom.com/placement-assistance
External URLs:
Telecom Gurukul: https://www.telecomgurukul.com
Reference URLs:
"Key Challenges in 5G Protocol Testing and Log Analysis" - Apeksha Telecom: https://www.apekshatelecom.com/5g-protocol-testing
Comments